Domain-Aware Neurosymbolic Agent (DANA) Architecture: Delivering Consistency & Accuracy for Industrial AI
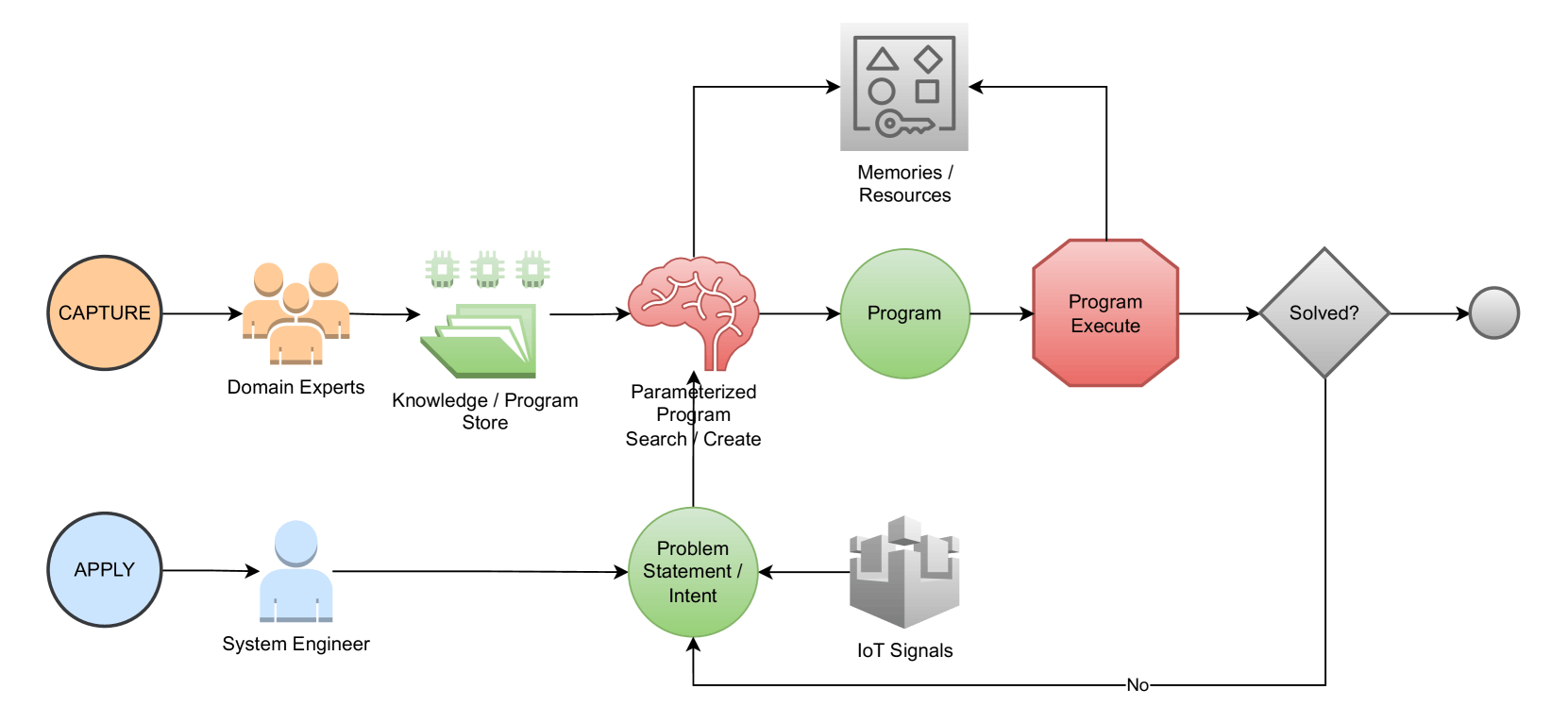
The Challenge: Industrial AI Consistency & Accuracy
As more and more global industrial enterprises join the AI Alliance, there emerges a substantial class of industrial AI applications in areas such as manufacturing, energy management and supply chain. While these AI use cases are diverse and growing in number, they share a common requirement: the ability to solve problems consistently and accurately.
This requirement poses a significant challenge for current generative AI tools. While LLMs and agents based on them are impressive in their flexibility and creativity, they fall far short when used to solve high-precision problems. A study in late 2023 found that such tools got it wrong over half of the times when analyzing SEC filings under realistic financial-analysis settings. Substitute financial analysis with heavy industrial manufacturing, where not only money but also human safety is at stake, and such performance would be abjectly unacceptable.
The Collaboration: Like-Minded Teams under AI Alliance’s Application Patterns & Tools Workgroup
AI Alliance members Aitomatic Inc. (based in Silicon Valley) and Fenrir Inc. (based in Japan) found strong common interests in solving such AI challenges in industrial fields. Aitomatic specializes in AI technology for physical industries such as semiconductor, energy and maritime, while Fenrir has for many years been building software powering some of Japan’s leading industrial enterprises such as Toyota and Kubota.
Further spurred by the discussions through AI Alliance’s Application Patterns & Tools workgroup – co-led by Adam Pingel and Amit Sangani – the Aitomatic and Fenrir teams embarked on a joint R&D effort over a better part of 2024 to find a new AI architecture that satisfies the stringent requirements of mission-critical industrial AI.
The Creation: Novel Domain-Aware Neurosymbolic Agent (DANA) Architecture
The DANA architecture directly addresses industrial AI’s need for consistency and accuracy by two fundamental innovations:
- A neurosymbolic AI system design which retains the power of neural networks in recognizing and analyzing complex patterns while assigning greater relative roles and responsibilities to symbolic structures and their more deterministic, precise execution; and
- A first-class treatment of domain expert knowledge, and systematic leveraging of such expertise in all aspects of the problem-solving process.
One variant of DANA has been implemented in Aitomatic’s OpenSSA framework.
The improvements are fantastic: with its architectural enhancements, DANA is able to not only achieve 90%+ accuracy on hard problems but also do so with superior consistency – performance levels unmatched by existing AI agent implementations.
The Pioneering Cases: Industrial Knowledge Capture and Scaling with DANA
DANA’s consistency and accuracy make it ideal for physical-industry workflows requiring precise AI analyses and recommendations.
Semiconductor
In semiconductor manufacturing, plasma etching recipe formulation is a critical process involving dozens of parameters that affect etch rate, selectivity, and uniformity. Suboptimal recipes can lead to inefficient cycle times and expensive quality problems like mask erosion, pattern distortion, and plasma instability, significantly impacting wafer yield. Traditionally, optimizing these recipes requires deep expertise and time-consuming analyses by scarce experts.
In this example, an Etching Advisor AI agent was constructed by integrating DANA with SemiKong, the world’s first open-source semiconductor LLM pioneered by the AI Alliance’s Foundation Models and Data workgroup. This agent demonstrates precise etching recipe analyses and recommendations, including pros-and-cons comparisons of feasible alternatives. Such an AI solution could help process engineers save time and quickly arrive at optimal recipes.
Marine
A global leader in marine electronics has a sprawling collection of sophisticated product designs and correspondingly complex equipment troubleshooting procedures. Among this company’s thousands of field engineers globally, there are a dozen or so veteran marine equipment experts who know the ins and outs of multiple product families and generations; these experts are highly in demand, but most of them are close to retiring, creating a real urgency in preserving their deep knowledge in this domain.
Thanks to having first-class consideration and treatment of human expertise, DANA is being used with success to capture and scale these experts’ experiential knowledge, such as:
- Important but uncommonly known facts about various regions’ different oceanic characteristics and conditions, which impact instrument sensitivity and how certain measurement data should be interpreted
- Inference rules about sonar image patterns and the under-water physical objects they usually correspond to; and
- Step-by-step and hierarchical procedures for troubleshooting malfunctioning equipment taking into account both product generations/lines and the physical operating environments
The DANA agents thus created are playing a crucial role in propagating experience accumulated over decades in the marine electronics field throughout the company’s multinational operations, delivering a multitude of benefits:
- Significantly reduced dependency on the small number of veteran experts;
- New insights from fusing knowledge across international engineering teams; and
- 3-4 times faster equipment issue resolution.
Railway Transportation
A leading railway operator in Japan, which serves millions of passengers daily, aims not only to keep its trains running on time but also to provide passengers with top-quality service experience throughout its station network. This requires effective integration of scheduling information with knowledge about regional cultural and taste differences.
With its integration of human knowledge with a wide array of informational resources including live data systems, DANA is being used to create monitoring and service-recommendation agents employed in this railway network. Use cases include:
- Culturally contextual guidance for visitors from other regions or countries
- Interest and budget-based travel recommendations
- Dynamic recommendations based on weather, special local events, and congestion information
The Coming Future of Industrial AI
As AI continues to proliferate in critical real-world industries, approaches like DANA that prioritize consistency, accuracy and domain-specific knowledge shall play an important role in building effective and trustworthy AI systems. And as more and more industrial enterprises join the AI Alliance’s movement of global, open AI collaboration, we have a unique opportunity to put DANA to work to enhance industrial efficiency and safety.
About the authors:
Kentaro Maegaito is the General Manager at Fenrir Inc.
Vinh Luong is the Head of Product Management at Aitomatic, Inc.